With the continuous construction and development of Extra-high-voltage (EHV, 230 ~ 800 KV) and Ultra-high-voltage (UHV, ≥800 KV) electricity transmission in China, online condition monitoring of power transmission and transformation equipment is playing an increasing important role in the safe and reliable operation of the power grid. Insulation defect fault is a major hidden danger of the power grid safety. The equipment and components in a high-voltage substation are permanently operated in a strong electric field. This can degrade the insulation material over time and – in an extreme case, if not repaired in time – may cause serious damage to such a plant.
Before the insulation defect failure of power equipment, there is generally a progressive partial discharge (PD) process. Insulation condition monitoring can be principally achieved through the detection of PD signals. Among the various PD detection approaches, spatially-coupled PD detection in the Very-high-frequency (VHF) and Ultra-high-frequency (UHF) band provides a promising means to non-intrusive and wide-coverage online condition monitoring of power equipment. The main challenge, however, is the interference identification in complex electromagnetic (EM) environment of power substations. This is extremely difficult under pure DC and AC & DC compound conditions (e.g. HVDC Converter), in which radiated PD signals are generally weak and sporadic pulses at random interval.
In this project a distributed high-precision & ultra-wide-band (UWB) spatially-coupled sensing system has been developed for substation partial discharge monitoring and localisation. The system is generally composed of three parts: UWB sensor, Smart data acquisition module, and Data analysis platform. The high-sensitivity featured UWB sensor allows the receival of very weak PD signal in complex substation EM environment. The Smart data acquisition module then synchronously samples the received EM signal in high-precision and extracts in real-time the RF pulses to dramatically reduce the data amount. This enables a continuous and undistorted preservation of the characteristics of broadband RF pulse waveform, thus allows the discrimination of PD signals and interference pulses at a later stage on the data analysis platform. On the data analysis platform, an advanced numerical procedure has been developed 1). to identify and localize the physical sources radiating the collected RF pulses; 2). to reduce noise and filter out undesired signals such as interferences; 3). to highlight potential sources of PD. The system can reduce the probability of an undetected insulation failure with significantly decreased maintenance expenses.
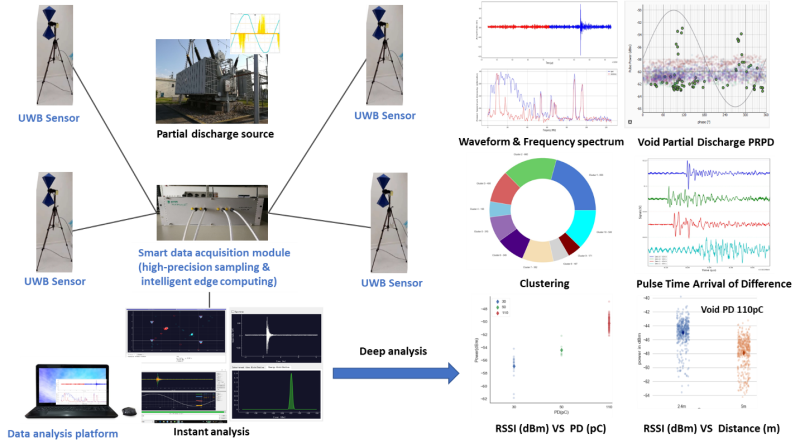
Research topics:
- Smart sensing for spatially-coupled PD detection, including ultra-wide-band antenna in the VHF/UHF band (50~800MHz) and Analog RF front end with high sensitivity (≤-70dBm) and wide dynamic signal receiving range (≥80dB)
- Edge intelligence for real-time RF pulse detection and instant RF pulse identification
- Machine learning for pulse signal clustering, feature extraction, PD and interference recognition, and noise reduction
- Received Signal Strength Indicator (RSSI) and Differential Time of Arrival (DTOA) combined PD source localisation
Related publications:
- [1] Benjamin Schubert, Jianguo Wei, Chandra Prakash Beura, Weilin Liu, Michael Beltle, "UHF-based Measurement of Partial Discharge in an Oil-filled Tank from Outside", VDE Fachtagung Hochspannungstechnik 2022, Berlin, Germany, 8-10 November 2022.
- [2] Jianguo Wei, Hui Huang, Yi Liu, Zhihao Yang, Benjamin Schubert, Weilin Liu, "Partial Discharge Measurement with Non-Intrusive Broadband RF Sensors in the Fabrication Inspection of an 800 kV HVDC Converter Transformer", 2022 IEEE International Conference on High Voltage Engineering and Application (ICHVE), Chongqing, China, September 25-29, 2022.
- [3] Mauro Palo, Benjamin Schubert, Jianguo Wei, Weilin Liu, Marcello Polenghi, Emanuele Ogliari, "Unsupervised Machine Learning for Blind Separation of Multiple PD Sources", 2022 IEEE 4th International Conference on Dielectrics (ICD), pp.197-201, 2022.
- [4] Andrea Matteri, Mauro Palo, Emanuele Ogliari, Benjamin Schubert, Jianguo Wei, Kai Gu, Weilin Liu, "Separation of radio-frequency signals triggered by valve switching in a HVDC converter by supervised machine learning methods", 2021 IEEE International Conference on Environment and Electrical Engineering and 2021 IEEE Industrial and Commercial Power Systems Europe (EEEIC / I&CPS Europe), pp.1-6, 2021.
- [5] Mauro Palo, Benjamin Schubert, Emanuele Ogliari, Jianguo Wei, Kai Gu, Weilin Liu, "Detection, Features Extraction and Classification of Radio-Frequency Pulses in a High-Voltage Power Substation: Results from a Measurement Campaign", 2020 IEEE 3rd International Conference on Dielectrics (ICD), pp.677-680, 2020.
- [6] Mauro Palo, Benjamin Schubert, Jianguo Wei, Weilin Liu, "Clustering-based Discrimination of multiple Partial Discharge Sources: A Case Study", 2019 IEEE Milan PowerTech, pp.1-6, 2019.
- [7] Benjamin Schubert, Jianguo Wei, Yong Zhang, Braulio Gomez Saavedra, Mauro Palo, and Weilin Liu, "A Distributed Data Acquisition System for Long-term Recording of Occasional Signals in the UHF Range," 2018 International Conference on Power System Technology (POWERCON), 2018, pp. 2984-2988, 2018.
- [8] Benjamin Schubert, Mauro Palo, and Thomas Schlechter, “Review of UHF-Based Signal Processing Approaches for Partial Discharge Detection”, Computer Aided Systems Theory – EUROCAST 2017. Lecture Notes in Computer Science, vol 10672. Springer, Cham. Jan. 2018, pp. 219-226.
- [9] Thomas Schlechter, Benjamin Schubert, and Mario Ludwig, “Review of UHF based Signal Processing Approaches for Partial Discharge Detection”, EUROCAST 2017, Computer Aided Systems Theory, Gran Canaria, Spain, Feb. 2017.